Revisiting Counterfactual Regression through the Lens of Gromov-Wasserstein Information Bottleneck
CoRR(2024)
摘要
As a promising individualized treatment effect (ITE) estimation method,
counterfactual regression (CFR) maps individuals' covariates to a latent space
and predicts their counterfactual outcomes. However, the selection bias between
control and treatment groups often imbalances the two groups' latent
distributions and negatively impacts this method's performance. In this study,
we revisit counterfactual regression through the lens of information bottleneck
and propose a novel learning paradigm called Gromov-Wasserstein information
bottleneck (GWIB). In this paradigm, we learn CFR by maximizing the mutual
information between covariates' latent representations and outcomes while
penalizing the kernelized mutual information between the latent representations
and the covariates. We demonstrate that the upper bound of the penalty term can
be implemented as a new regularizer consisting of i) the fused
Gromov-Wasserstein distance between the latent representations of different
groups and ii) the gap between the transport cost generated by the model and
the cross-group Gromov-Wasserstein distance between the latent representations
and the covariates. GWIB effectively learns the CFR model through alternating
optimization, suppressing selection bias while avoiding trivial latent
distributions. Experiments on ITE estimation tasks show that GWIB consistently
outperforms state-of-the-art CFR methods. To promote the research community, we
release our project at https://github.com/peteryang1031/Causal-GWIB.
更多查看译文
AI 理解论文
溯源树
样例
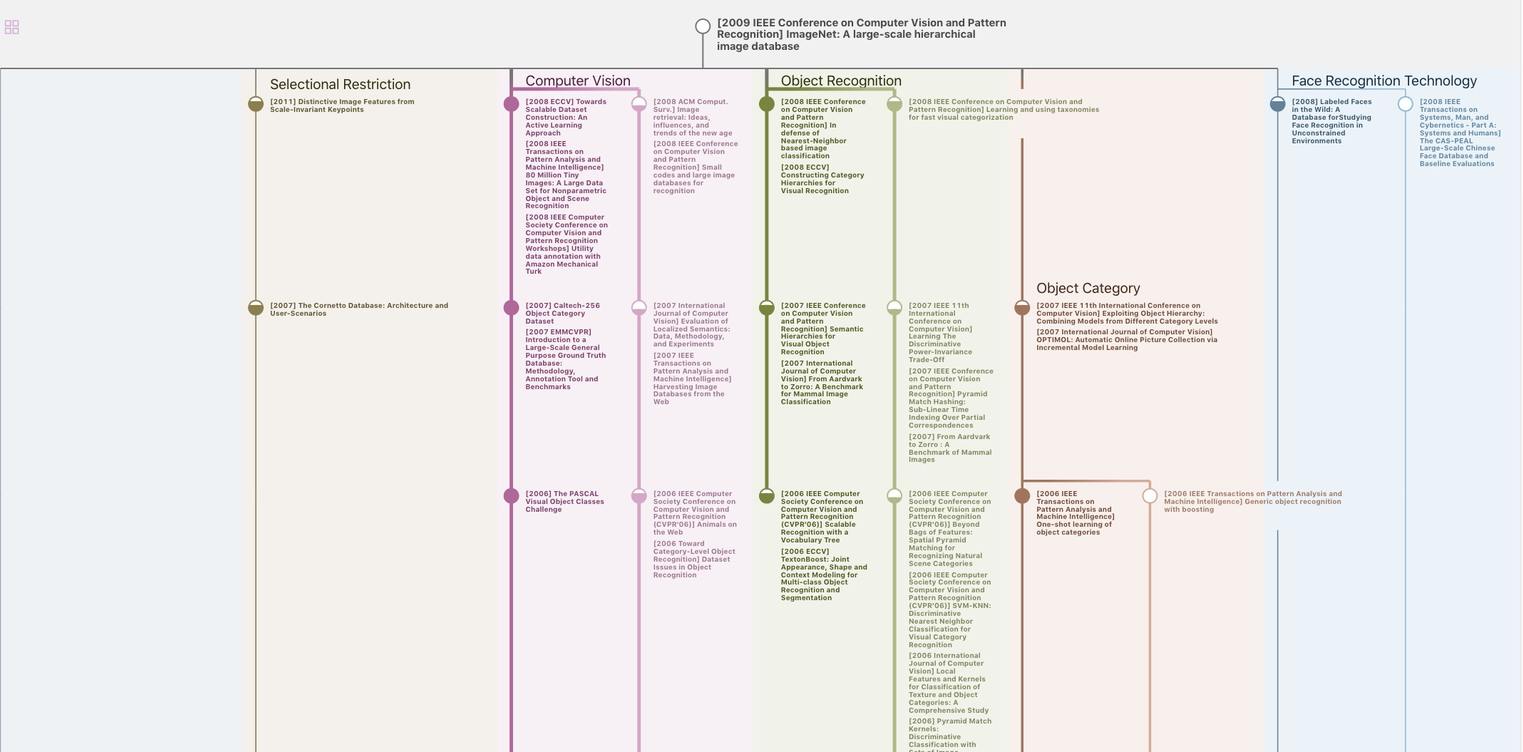
生成溯源树,研究论文发展脉络
Chat Paper
正在生成论文摘要