Automatic Data Curation for Self-Supervised Learning: A Clustering-Based Approach
CoRR(2024)
摘要
Self-supervised features are the cornerstone of modern machine learning
systems. They are typically pre-trained on data collections whose construction
and curation typically require extensive human effort. This manual process has
some limitations similar to those encountered in supervised learning, e.g., the
crowd-sourced selection of data is costly and time-consuming, preventing
scaling the dataset size. In this work, we consider the problem of automatic
curation of high-quality datasets for self-supervised pre-training. We posit
that such datasets should be large, diverse and balanced, and propose a
clustering-based approach for building ones satisfying all these criteria. Our
method involves successive and hierarchical applications of k-means on a
large and diverse data repository to obtain clusters that distribute uniformly
among data concepts, followed by a hierarchical, balanced sampling step from
these clusters. Extensive experiments on three different data domains including
web-based images, satellite images and text show that features trained on our
automatically curated datasets outperform those trained on uncurated data while
being on par or better than ones trained on manually curated data.
更多查看译文
AI 理解论文
溯源树
样例
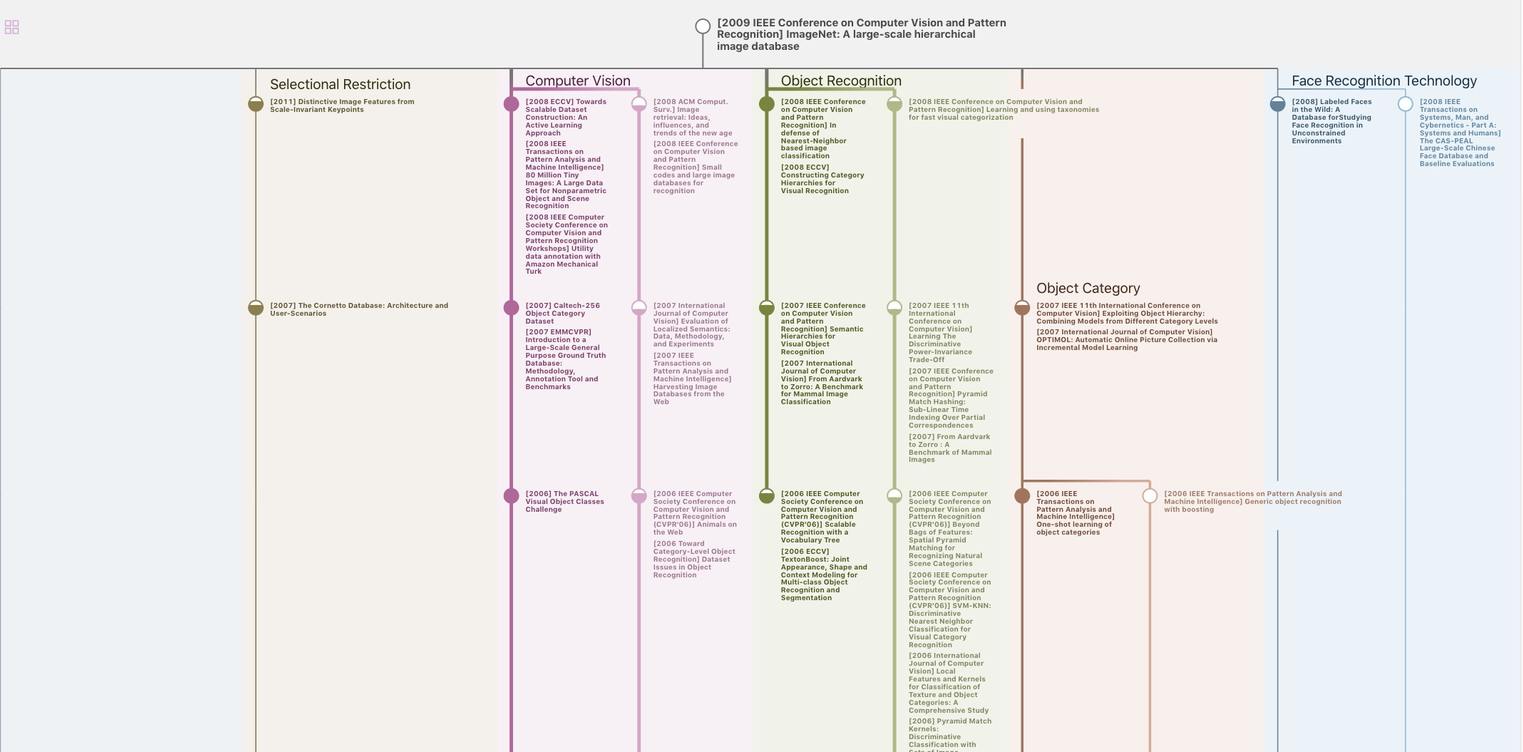
生成溯源树,研究论文发展脉络
Chat Paper
正在生成论文摘要