Group Fairness via Group Consensus.
ACM Conference on Fairness, Accountability and Transparency(2024)
摘要
Ensuring equitable impact of machine learning models across different societal groups is of utmost importance for real-world machine learning applications. Prior research in fairness has predominantly focused on adjusting model outputs through pre-processing, in-processing, or post-processing techniques. These techniques focus on correcting bias in either the data or the model. However, we argue that the bias in the data and model should be addressed in conjunction. To achieve this, we propose an algorithm called GroupDebias to reduce unfairness in the data in a model-guided fashion, thereby enabling models to exhibit more equitable behavior. Even though it is model-aware, the core idea of GroupDebias is independent of the model architecture, making it a versatile and effective approach that can be broadly applied across various domains and model types. Our method focuses on systematically addressing biases present in the training data itself by adaptively dropping samples that increase the biases in the model. Theoretically, the proposed approach enjoys a guaranteed improvement in demographic parity at the expense of a bounded reduction in balanced accuracy. A comprehensive evaluation of the GroupDebias algorithm through extensive experiments on diverse datasets and machine learning models demonstrates that GroupDebias consistently and significantly outperforms existing fairness enhancement techniques, achieving a more substantial reduction in unfairness with minimal impact on model performance.
更多查看译文
AI 理解论文
溯源树
样例
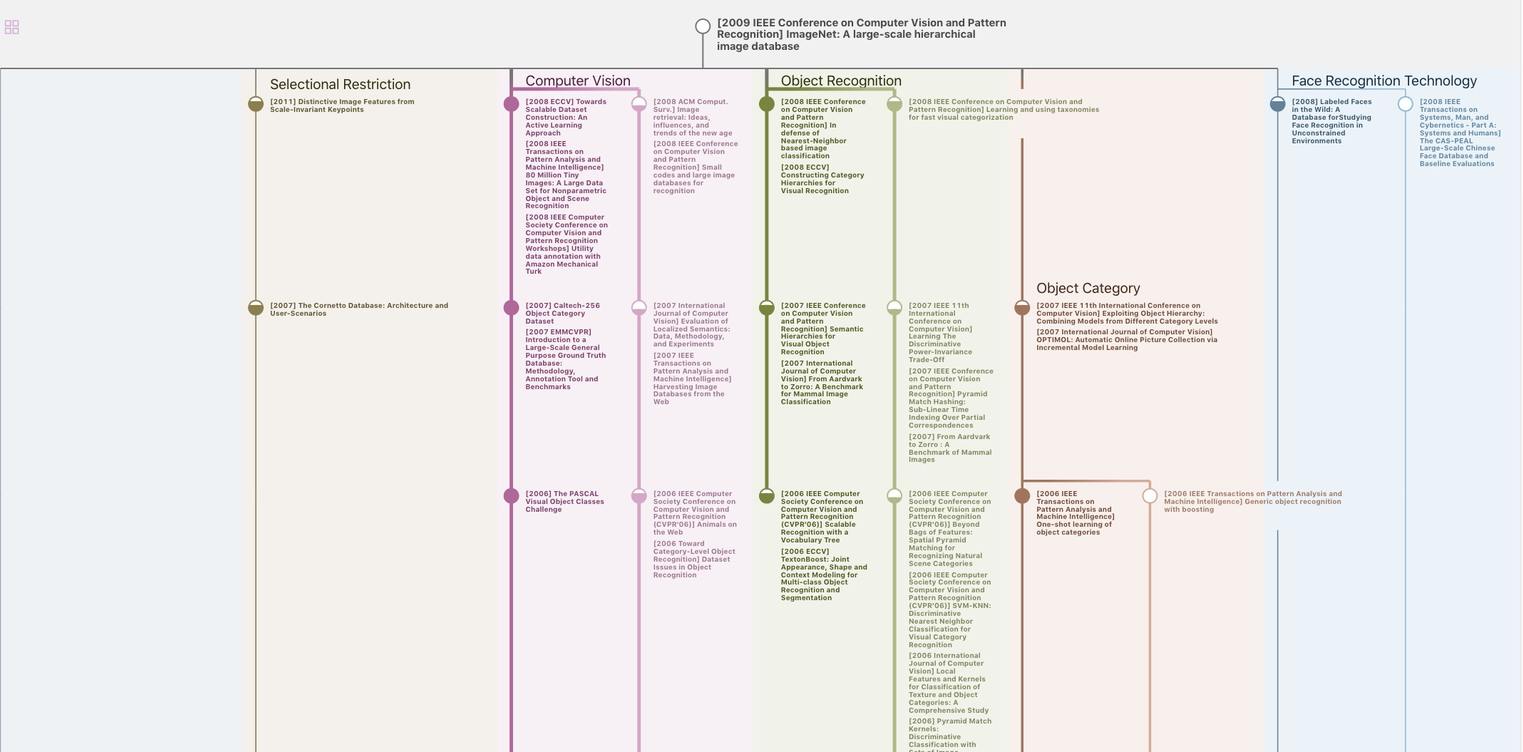
生成溯源树,研究论文发展脉络
Chat Paper
正在生成论文摘要