LLMs Are Not Intelligent Thinkers: Introducing Mathematical Topic Tree Benchmark for Comprehensive Evaluation of LLMs
CoRR(2024)
摘要
Large language models (LLMs) demonstrate impressive capabilities in
mathematical reasoning. However, despite these achievements, current
evaluations are mostly limited to specific mathematical topics, and it remains
unclear whether LLMs are genuinely engaging in reasoning. To address these
gaps, we present the Mathematical Topics Tree (MaTT) benchmark, a challenging
and structured benchmark that offers 1,958 questions across a wide array of
mathematical subjects, each paired with a detailed hierarchical chain of
topics. Upon assessing different LLMs using the MaTT benchmark, we find that
the most advanced model, GPT-4, achieved a mere 54% accuracy in a
multiple-choice scenario. Interestingly, even when employing Chain-of-Thought
prompting, we observe mostly no notable improvement. Moreover, LLMs accuracy
dramatically reduced by up to 24.2 percentage point when the questions were
presented without providing choices. Further detailed analysis of the LLMs'
performance across a range of topics showed significant discrepancy even for
closely related subtopics within the same general mathematical area. In an
effort to pinpoint the reasons behind LLMs performances, we conducted a manual
evaluation of the completeness and correctness of the explanations generated by
GPT-4 when choices were available. Surprisingly, we find that in only 53.3% of
the instances where the model provided a correct answer, the accompanying
explanations were deemed complete and accurate, i.e., the model engaged in
genuine reasoning.
更多查看译文
AI 理解论文
溯源树
样例
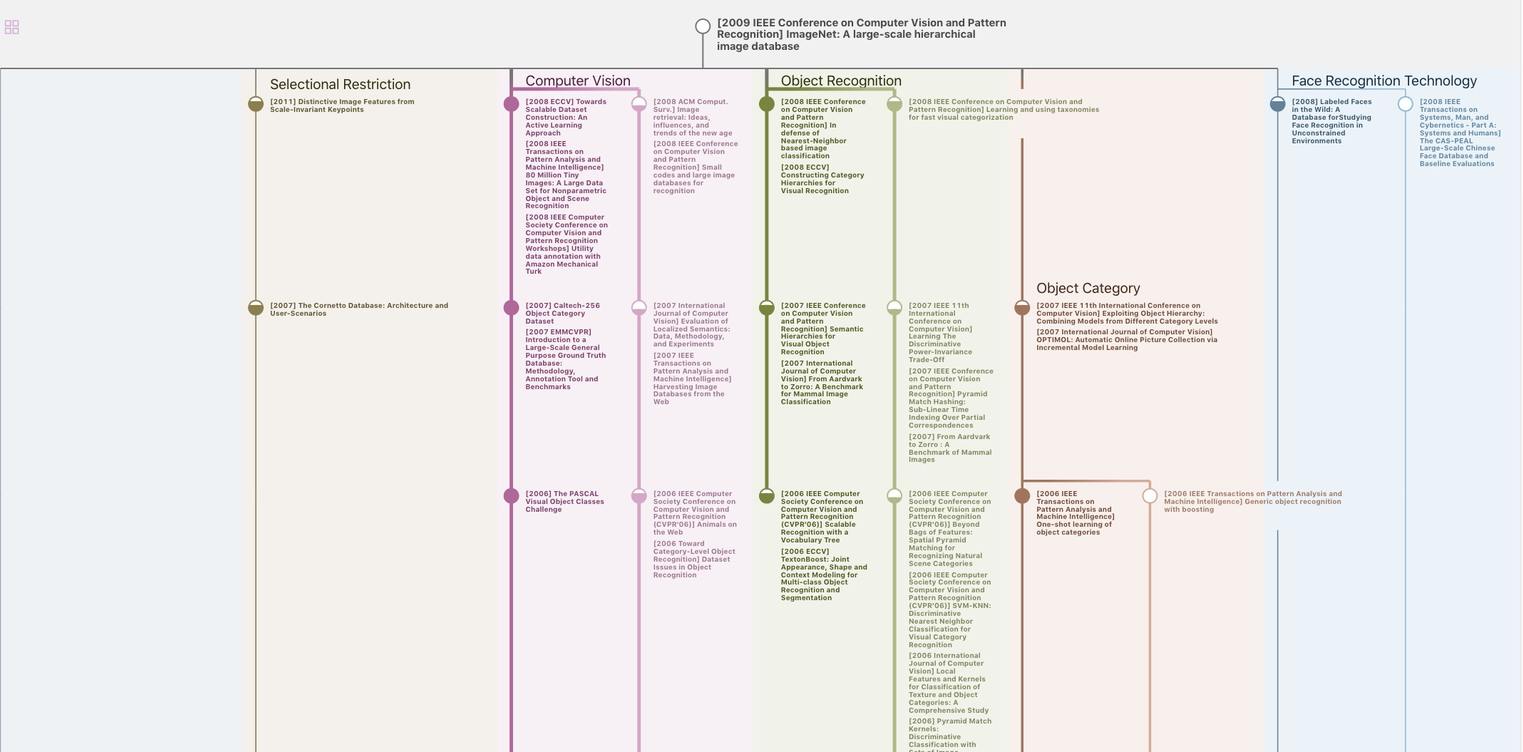
生成溯源树,研究论文发展脉络
Chat Paper
正在生成论文摘要