When Do Skills Help Reinforcement Learning? A Theoretical Analysis of Temporal Abstractions
ICML(2024)
摘要
Skills are temporal abstractions that are intended to improve reinforcementlearning (RL) performance through hierarchical RL. Despite our intuition aboutthe properties of an environment that make skills useful, a precisecharacterization has been absent. We provide the first such characterization,focusing on the utility of deterministic skills in deterministic sparse-rewardenvironments with finite action spaces. We show theoretically and empiricallythat RL performance gain from skills is worse in environments where solutionsto states are less compressible. Additional theoretical results suggest thatskills benefit exploration more than they benefit learning from existingexperience, and that using unexpressive skills such as macroactions may worsenRL performance. We hope our findings can guide research on automatic skilldiscovery and help RL practitioners better decide when and how to use skills.
更多查看译文
AI 理解论文
溯源树
样例
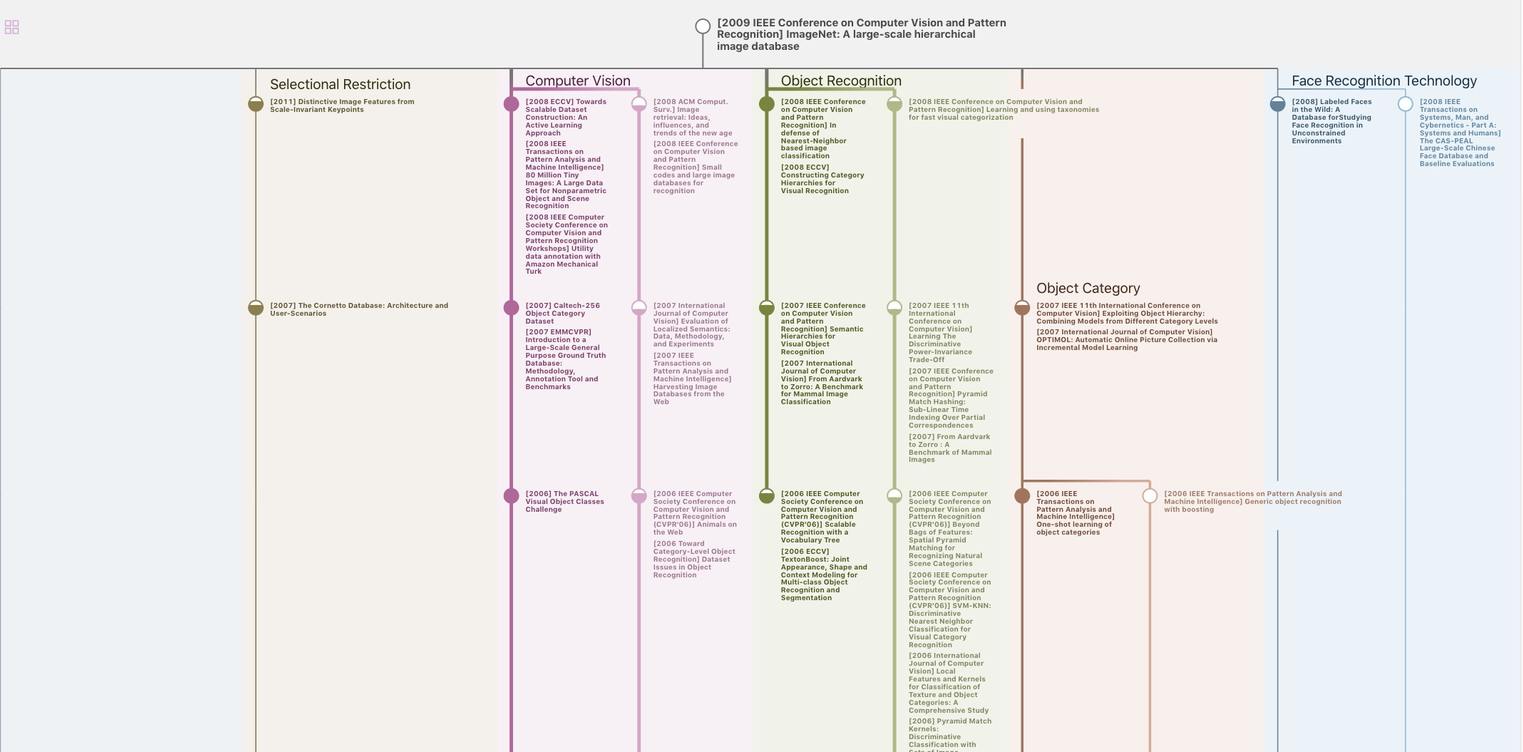
生成溯源树,研究论文发展脉络
Chat Paper
正在生成论文摘要