Enhancing Wearable Based Real-Time Glucose Monitoring Via Phasic Image Representation Learning Based Deep Learning
arxiv(2024)
摘要
In the U.S., over a third of adults are pre-diabetic, with 80% unaware of their status. This underlines the need for better glucose monitoring to prevent type 2 diabetes and related heart diseases. Existing wearable glucose monitors are limited by the lack of models trained on small datasets, as collecting extensive glucose data is often costly and impractical. Our study introduces a novel machine learning method using modified recurrence plots in the frequency domain to improve glucose level prediction accuracy from wearable device data, even with limited datasets. This technique combines advanced signal processing with machine learning to extract more meaningful features. We tested our method against existing models using historical data, showing that our approach surpasses the current 87% accuracy benchmark in predicting real-time interstitial glucose levels.
更多查看译文
AI 理解论文
溯源树
样例
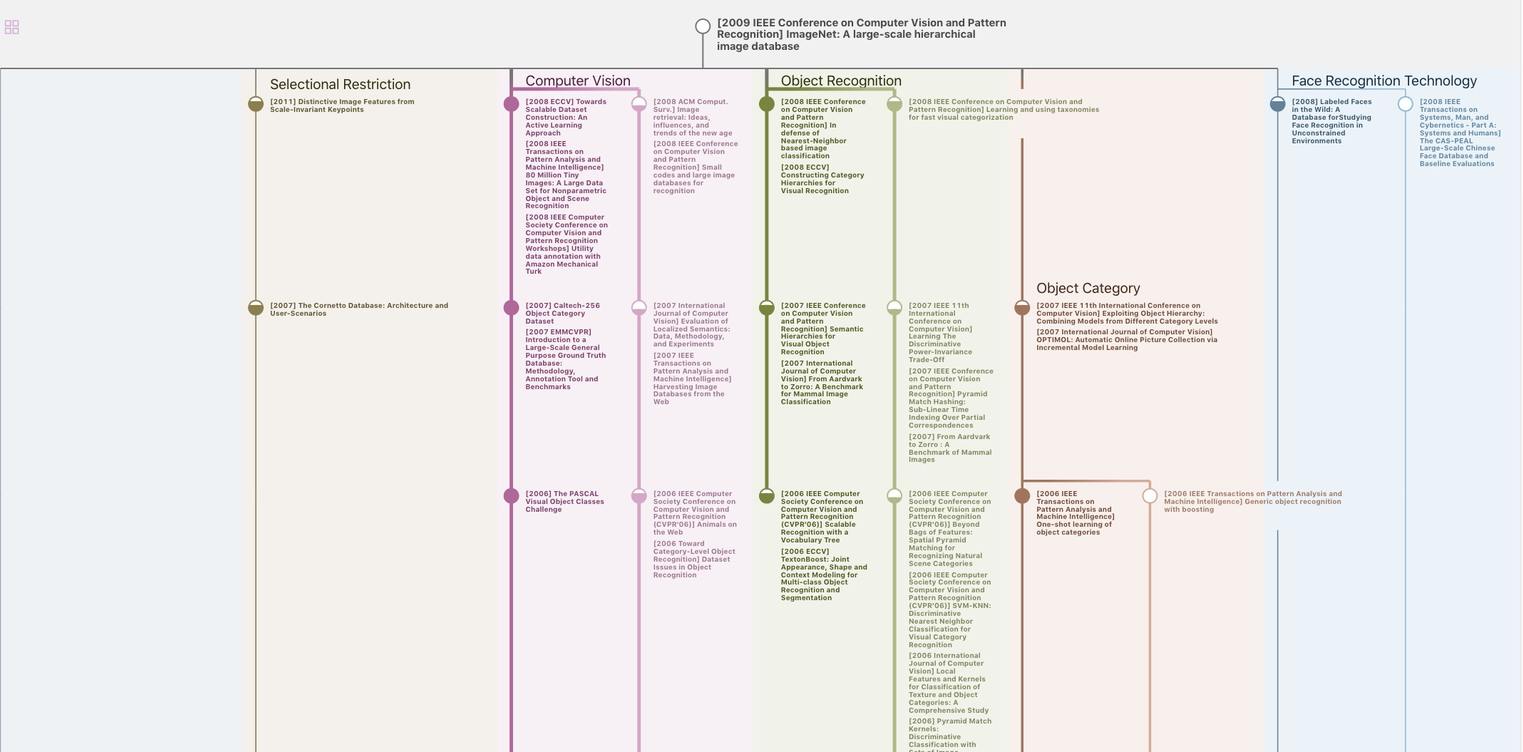
生成溯源树,研究论文发展脉络
Chat Paper
正在生成论文摘要