Performance evaluation of multivariate deep-time convolution neural architectures for short-term electricity forecasting: Findings and failures
Energy 360(2024)
摘要
Deep learning (DL) models hold great promise in enhancing the decision-making abilities of electricity market participants and system operators in the short term, as they excel at capturing the intricate interdependencies and uncertainties in electricity consumption. Nevertheless, currently, the adoption of DL models for electricity prediction is not keeping up with the advancements in DL research. Within this framework, the aims of this study are to examine the probabilistic, multivariate, and multihorizon time series prediction of deep time convolution neural (DTCN) models. The effectiveness of DTCN in forecasting electricity consumption over daily, weekly and monthly time horizons is emperically assessed. We analyze both probabilistic and deterministic predictions. The evaluation data comprises real residential electricity consumption, average temperature and humidity with hourly granularity, collected over one year from a residential building. For evaluation, we considered accuracy and uncertainties, sensitivity to hyperparameters, the impact of exogeneous variables, as well as computational efficiency. Results show that the probabilistic models offer greater advantages when it comes to forecasting on multihorizons, especially for diverse datasets with non-uniform time series models. Unlike traditional models, the DTCN models demonstrated superior performance in capturing complex patterns and reducing forecast errors in short-term scenarios. However, their performance can be influenced by the sensitivity of hyperparameters. These findings can act as a benchmark for quantitatively assessing the performance of novel multivariate DL models in predicting electricity demand.
更多查看译文
关键词
Deep learning,Electricity forecasting,Time series,Performance evaluation,Multivariate modelling
AI 理解论文
溯源树
样例
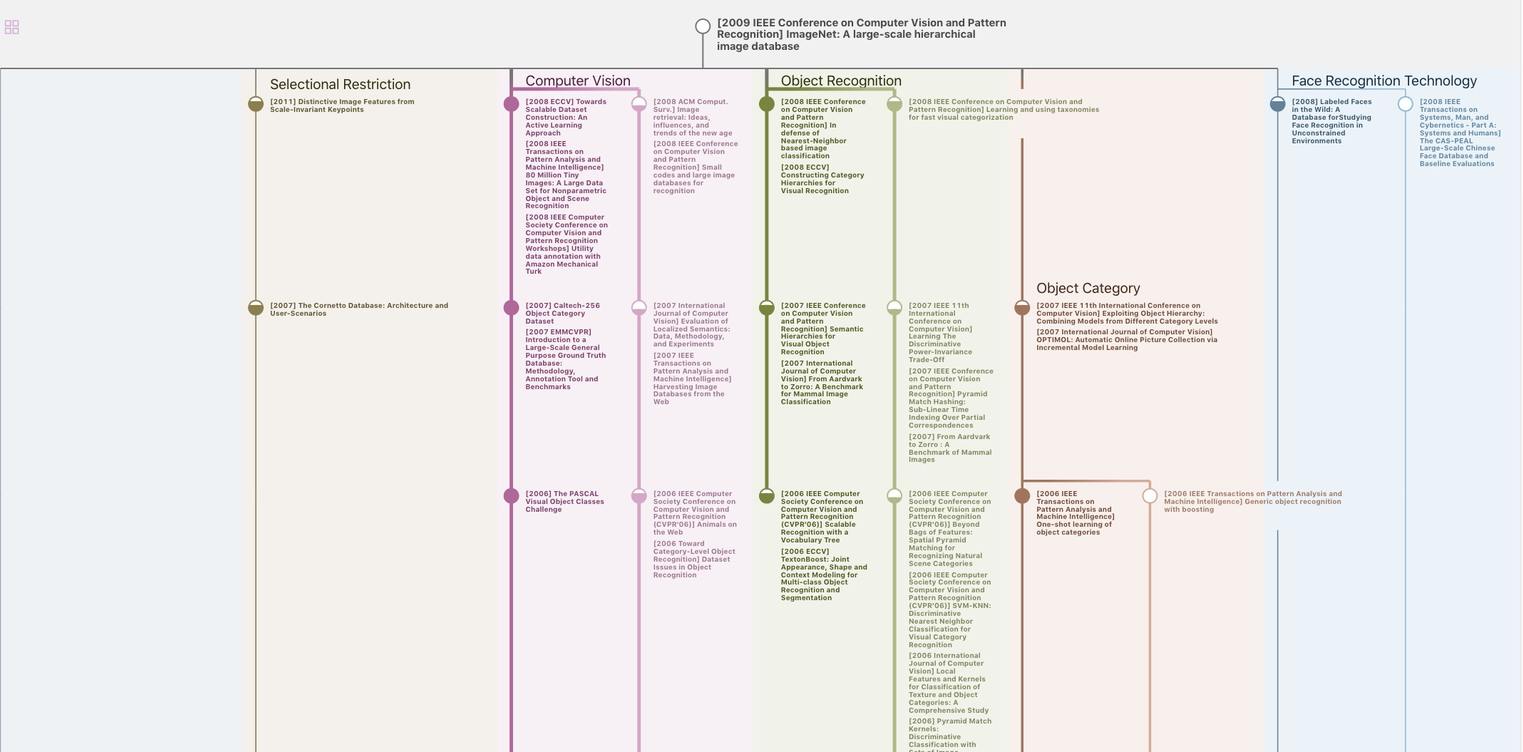
生成溯源树,研究论文发展脉络
Chat Paper
正在生成论文摘要