Accurate Subtyping of Lung Cancers by Modelling Class Dependencies
ISBI(2024)
Abstract
Identifying subtypes and histological patterns is crucial for lung cancer diagnosis and treatment. Nevertheless, datasets with complete subtyping annotations are scarce, and most existing work primarily focuses on categorising lung cancers into fundamental types, omitting the distinction of adenocarcinoma patterns. We present a computational approach for a more comprehensive lung cancer subtyping from histology by modelling the dependencies between cancer subtypes and histological patterns in a multi-label setting. Our approach utilises slide-level labels indicating cancer subtypes as well as the presence of cancer-associated patterns, thereby alleviating the need for labour-intensive region-based annotations. A new dataset with cancer-associated pattern labels is constructed and combined with publicly available datasets. We evaluate our model’s ability to simultaneously differentiate cancer subtypes and cancer-associated patterns. The result demonstrates that our modules enable conventional weakly-supervised classification models on multi-label problems, achieving subset accuracy of 84% when differentiating lung cancer subtypes and cancer-associated histological patterns. Code: https://github.com/GeorgeBatch/dependency-mil
MoreTranslated text
Key words
lung cancer,computational pathology,multi-label classification,multiple-instance learning
AI Read Science
Must-Reading Tree
Example
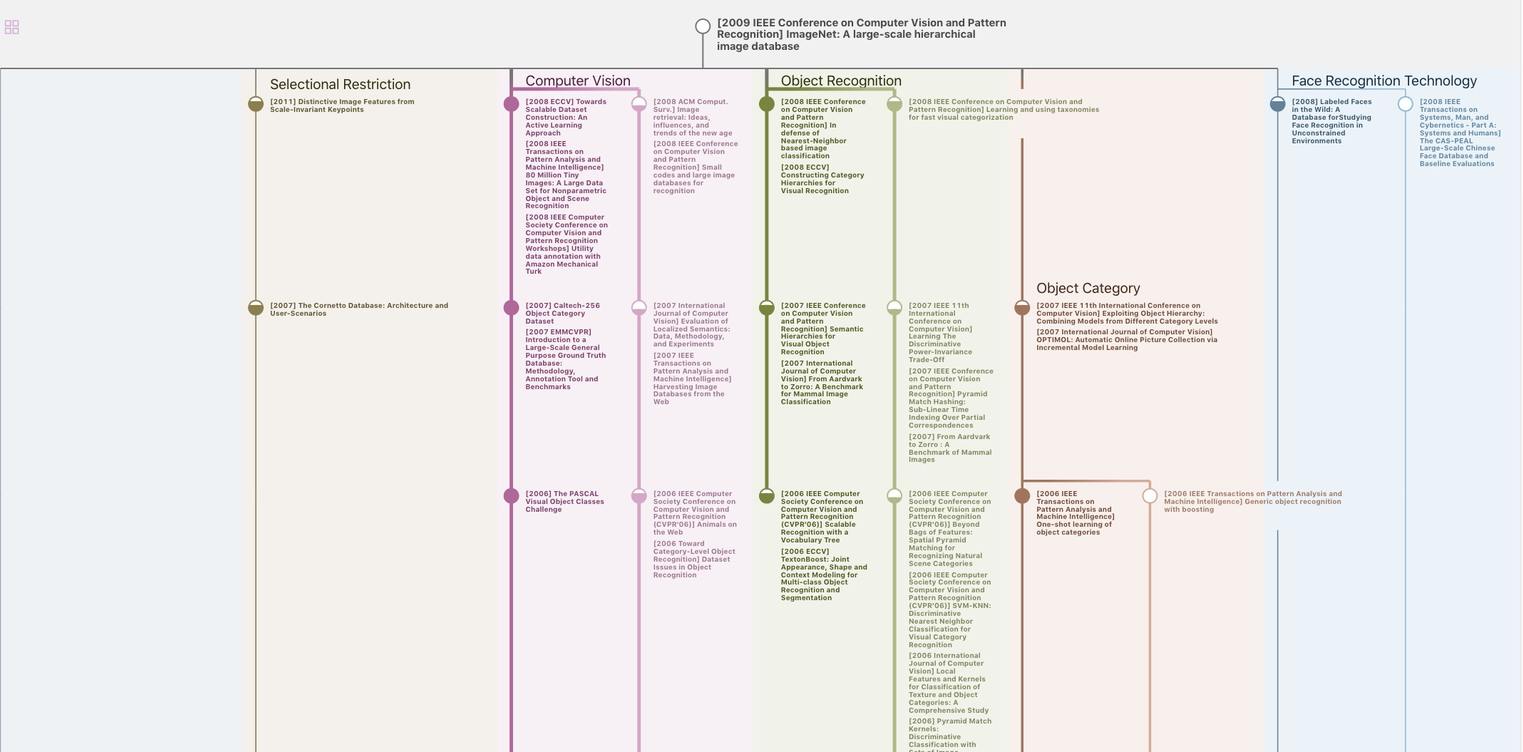
Generate MRT to find the research sequence of this paper
Chat Paper
Summary is being generated by the instructions you defined