Can Algorithms Replace Expert Knowledge for Causal Inference? A Case Study on Novice Use of Causal Discovery.
American journal of epidemiology(2024)
摘要
BACKGROUND:With growing interest in causal inference and machine learning among epidemiologists, there is increasing discussion of causal discovery algorithms for guiding covariate selection. We present a case study of novice application of causal discovery tools and attempt to validate the results against a well-established causal relationship. METHODS:As a case study, we attempted causal discovery of relationships relevant to the effect of adherence on mortality in the placebo arm of the Coronary Drug Project (CDP) dataset. We used four algorithms available as existing software implementations and varied several model inputs. RESULTS:We identified 15 adjustment sets from 17 model parameterizations. When applied to a baseline covariate adjustment analysis, these 15 adjustment sets returned effect estimates with similar magnitude and direction of bias as prior published results. When using methods to control for time-varying confounding, there was generally more residual bias than compared to expert-selected adjustment sets. CONCLUSION:Although causal discovery algorithms can perform on par with expert knowledge, we do not recommend novice use of causal discovery without the input of experts in causal discovery. Expert support is recommended to aid in choosing the algorithm, selecting input parameters, assessing underlying assumptions, and finalizing selection of the adjustment variables.
更多查看译文
AI 理解论文
溯源树
样例
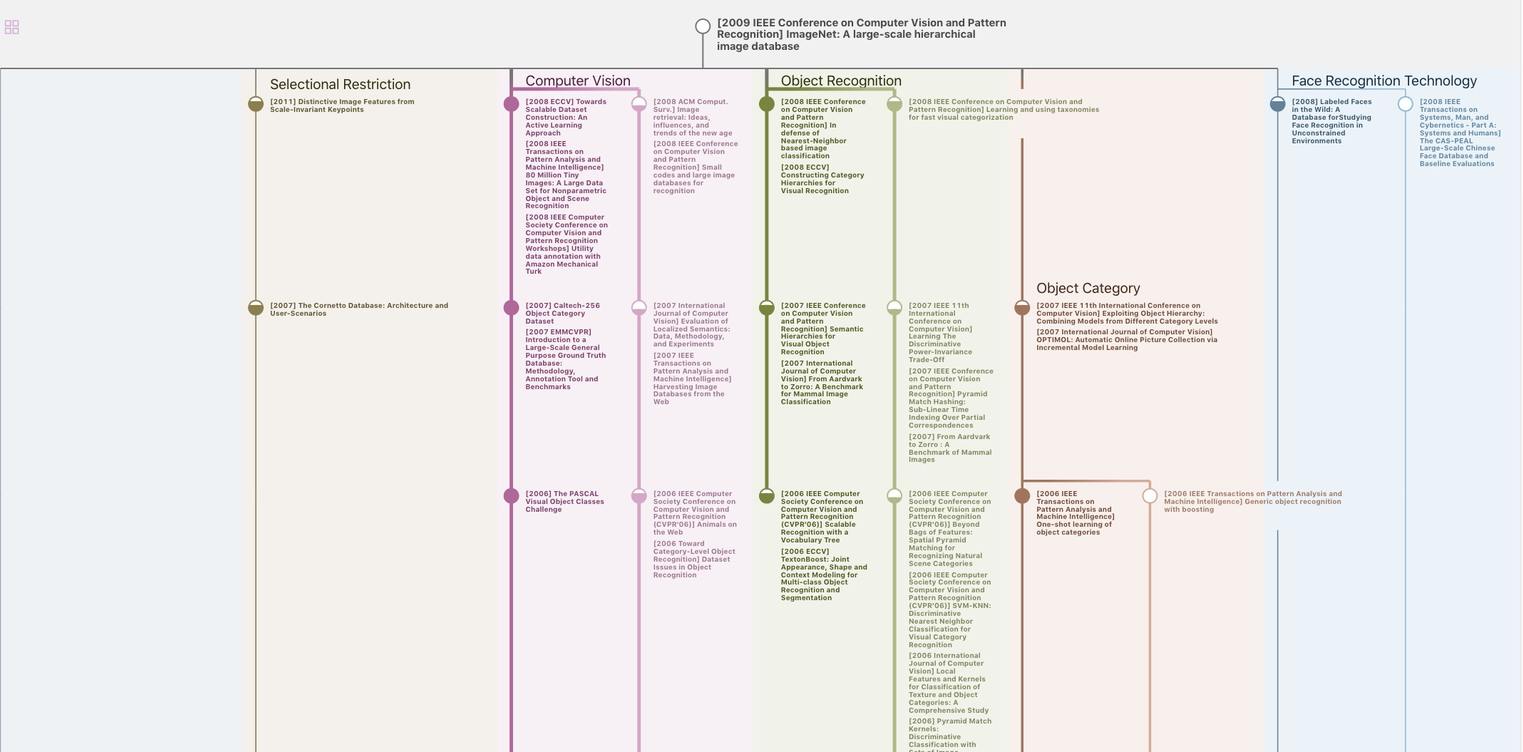
生成溯源树,研究论文发展脉络
Chat Paper
正在生成论文摘要