Single Domain Generalization for Scene Classification Using Style-Oriented Data Augmentation
IGARSS 2024 - 2024 IEEE International Geoscience and Remote Sensing Symposium(2024)
摘要
Domain generalization (DG), which tries to improve the performance of models trained with known domains but applied to unknown domains, is an important step towards practical solutions in real-world scenarios. In this paper, we tackle a much more difficult scenario called single domain generation in scene classification problem, where only one source domain is available during training. Existing DG methods usually focus on extracting invariant features from different known domains and often suffer from overfitting issues. Therefore, to tackle the above challenge, we propose a randomly-stylized data augmentation method, which enables randomized style perturbation of the training data, to alleviate the overfitting problem and to improve the robustness of the resulting model. On a multidomain scene classification benchmark, our method achieves an accuracy improvement of 0.4%-2.5% compared to other DG methods.
更多查看译文
关键词
Domain Generalization,Scene Classification,Stylization
AI 理解论文
溯源树
样例
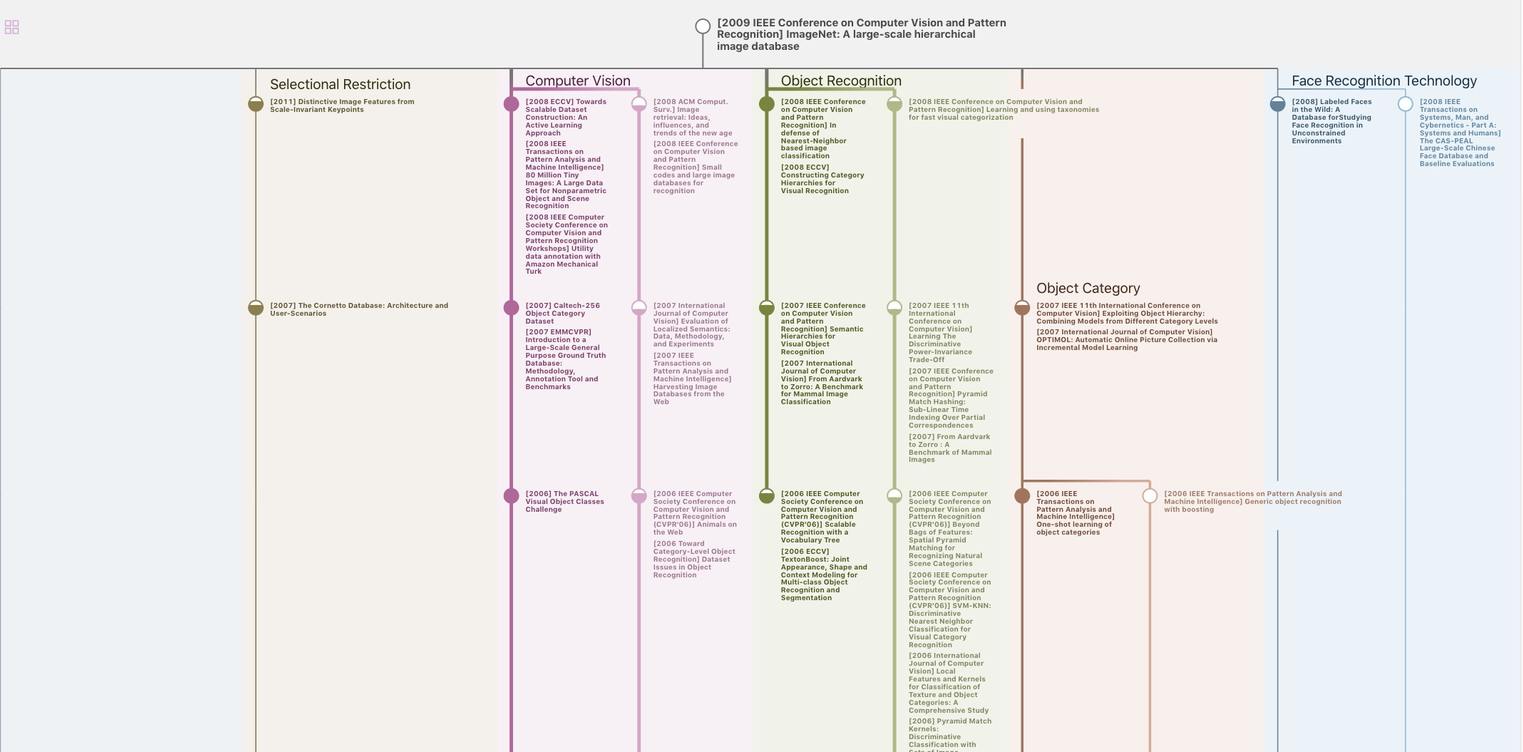
生成溯源树,研究论文发展脉络
Chat Paper
正在生成论文摘要