MEFSR-GAN: A Multi-Exposure Feedback and Super-Resolution Multitask Network Via Generative Adversarial Networks
REMOTE SENSING(2024)
摘要
In applications such as satellite remote sensing and aerial photography, imaging equipment must capture brightness information of different ground scenes within a restricted dynamic range. Due to camera sensor limitations, captured images can represent only a portion of such information, which results in lower resolution and lower dynamic range compared with real scenes. Image super resolution (SR) and multiple-exposure image fusion (MEF) are commonly employed technologies to address these issues. Nonetheless, these two problems are often researched in separate directions. In this paper, we propose MEFSR-GAN: an end-to-end framework based on generative adversarial networks that simultaneously combines super-resolution and multiple-exposure fusion. MEFSR-GAN includes a generator and two discriminators. The generator network consists of two parallel sub-networks for under-exposure and over-exposure, each containing a feature extraction block (FEB), a super-resolution block (SRB), and several multiple-exposure feedback blocks (MEFBs). It processes low-resolution under- and over-exposed images to produce high-resolution high dynamic range (HDR) images. These images are evaluated by two discriminator networks, driving the generator to generate realistic high-resolution HDR outputs through multi-goal training. Extensive qualitative and quantitative experiments were conducted on the SICE dataset, yielding a PSNR of 24.821 and an SSIM of 0.896 for 2× upscaling. These results demonstrate that MEFSR-GAN outperforms existing methods in terms of both visual effects and objective evaluation metrics, thereby establishing itself as a state-of-the-art technology.
更多查看译文
关键词
multitask networks,super resolution,multiple exposure fusion,generative adversarial networks
AI 理解论文
溯源树
样例
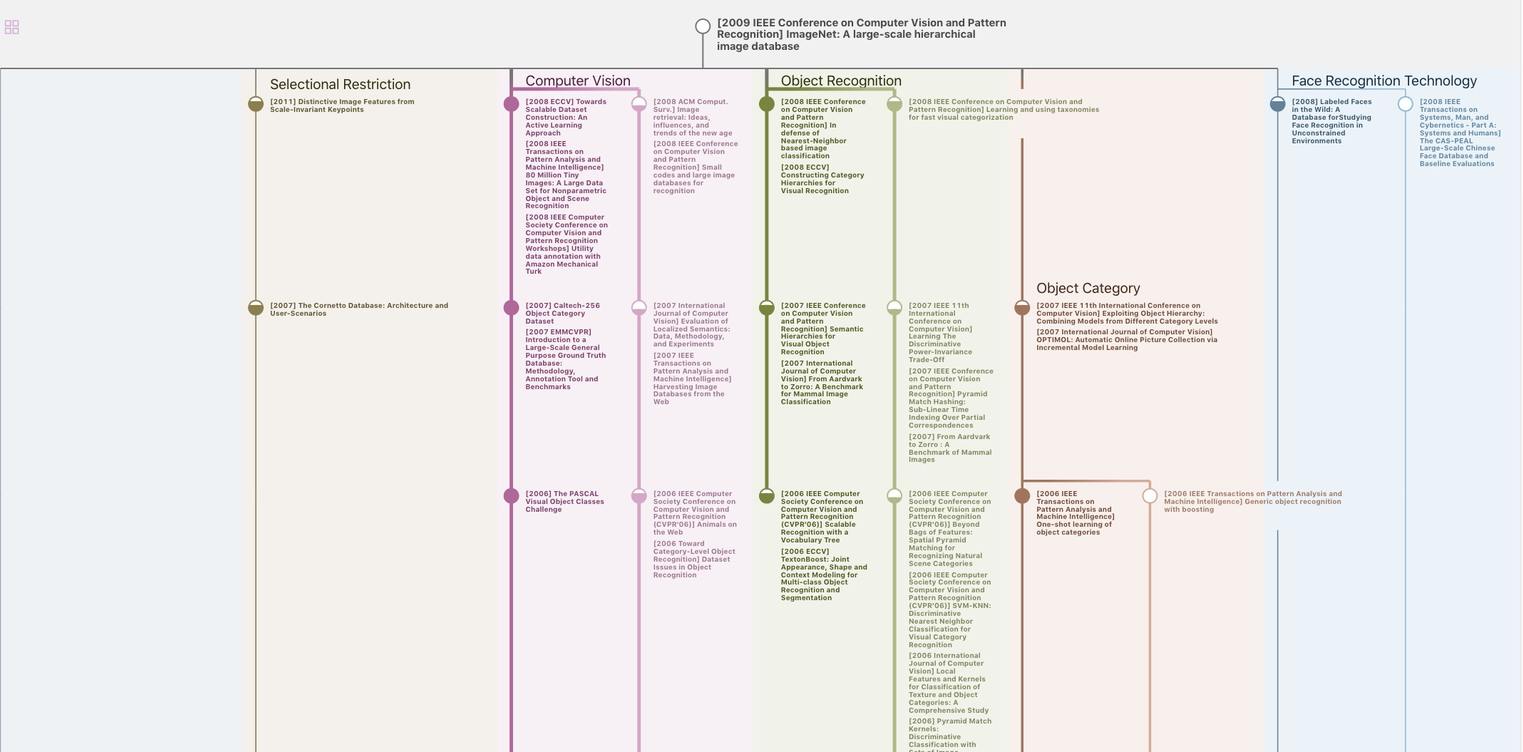
生成溯源树,研究论文发展脉络
Chat Paper
正在生成论文摘要