Lens Modeling of STRIDES Strongly Lensed Quasars Using Neural Posterior Estimation
arxiv(2024)
摘要
Strongly lensed quasars can be used to constrain cosmological parameters through time-delay cosmography. Models of the lens masses are a necessary component of this analysis. To enable time-delay cosmography from a sample of 𝒪(10^3) lenses, which will soon become available from surveys like the Rubin Observatory's Legacy Survey of Space and Time (LSST) and the Euclid Wide Survey, we require fast and standardizable modeling techniques. To address this need, we apply neural posterior estimation (NPE) for modeling galaxy-scale strongly lensed quasars from the Strong Lensing Insights into the Dark Energy Survey (STRIDES) sample. NPE brings two advantages: speed and the ability to implicitly marginalize over nuisance parameters. We extend this method by employing sequential NPE to increase precision of mass model posteriors. We then fold individual lens models into a hierarchical Bayesian inference to recover the population distribution of lens mass parameters, accounting for out-of-distribution shift. After verifying our method using simulated analogs of the STRIDES lens sample, we apply our method to 14 Hubble Space Telescope single-filter observations. We find the population mean of the power-law elliptical mass distribution slope, γ_lens, to be ℳ_γ_lens=2.13 ± 0.06. Our result represents the first population-level constraint for these systems. This population-level inference from fully automated modeling is an important stepping stone towards cosmological inference with large samples of strongly lensed quasars.
更多查看译文
AI 理解论文
溯源树
样例
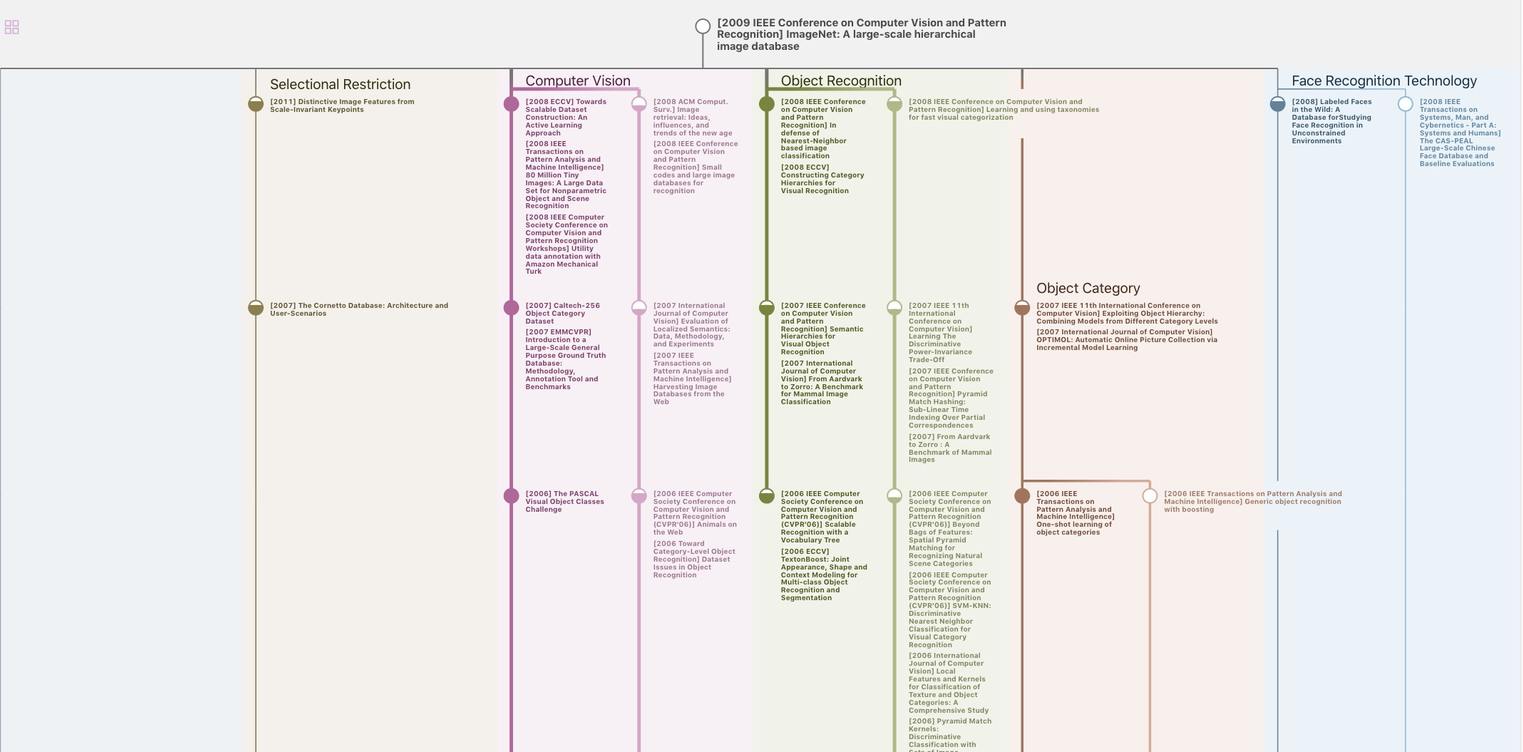
生成溯源树,研究论文发展脉络
Chat Paper
正在生成论文摘要